
For this purpose, 63 layers of CNN-centered deep architecture are intended for feature acquisition. In this work, a deep feature extraction methodology is presented for suspicious activity recognition to tackle the above-mentioned challenges. The following are some of the most significant challenges: (a) occlusion, (b) illumination, (c) variations in objects size, (d) variations in appearances because of varying clothing, (e) the computational time is another major challenge, and (f) the students may help each other by sharing pen or white paper, which makes the issue of distinguishing very hard to decide. Aside from its significance, the activity detection may worsen due to several technical obstacles. Automating the surveillance events, reduce the invigilators' workload during an examination. (a) Simultaneous activities where individuals can complete a few activities simultaneously, like using scientific calculator for engineering students, using drawing equipment, (b) some times prediction ambiguity occurs, for example, front watching to copy from students who are in front of him or her can be related to normal front watching, (c) intraclass similarity, where students wear uniform cloth,(d) lack of lightning, (e) camera focus which is difficult to handle. A deep learning algorithm can detect such behaviors automatically. HAR has the potential to have a significant impact on a variety of human activities. In addition, it limits the biases of invigilators while taking corrective measurements. A good Activity prediction can help the invigilator to take action for misbehaving of students in the exam. These activities demand a smart framework that can capable of issuing a warning or alarm as a result. Suspicious acts such as Back watching, Front watching, showing gestures, Side watching, Answer sharing, copying from notes, carrying a smartphone Walking in the exam hall warrant immediate attention. To handle student suspicious activity in exam student cheating detection systems is in significant demand in universities during this COVID_19 pandemic to ensure both safeties of the invigilators and students. For example, HAR is an application in the airport to detect passengers' activity around boarding areas, baggage claim, passenger arrival places, city buses, university gates, and even in some cafeterias to recognize fighting, item lost, theft, vandalism, and the likes.
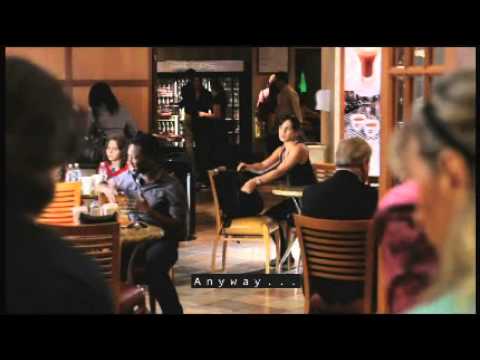
Now a day's HAR is widely used in every sector where security is the primary concern for the organization. 2019), where it plays two significant roles: health care of the elderly and disabled and adaptation of the environment to the residents’ habits to improve the quality of their lives (Ternes et al. Firstly, HAR is widely used in the area of smart homes (Du et al. We, as humans, possess an amazing skill to comprehend information that others pass on through their movements like the gesture of a certain body part or the motion of the entire body(Feng et al. Academic cheating is usually administratively handled at the classroom or institutional level(Miller et al. The result indicates the suggested frameworks soundness.Įxaminations are a fundamental part of any education program. The suggested model was further tested on the CIFAR-100 dataset, and it was shown to be accurate to the tune of 0.89796. With a performance of 0.9299 in terms of accuracy, the cubic SVM gets the greatest efficiency scores.
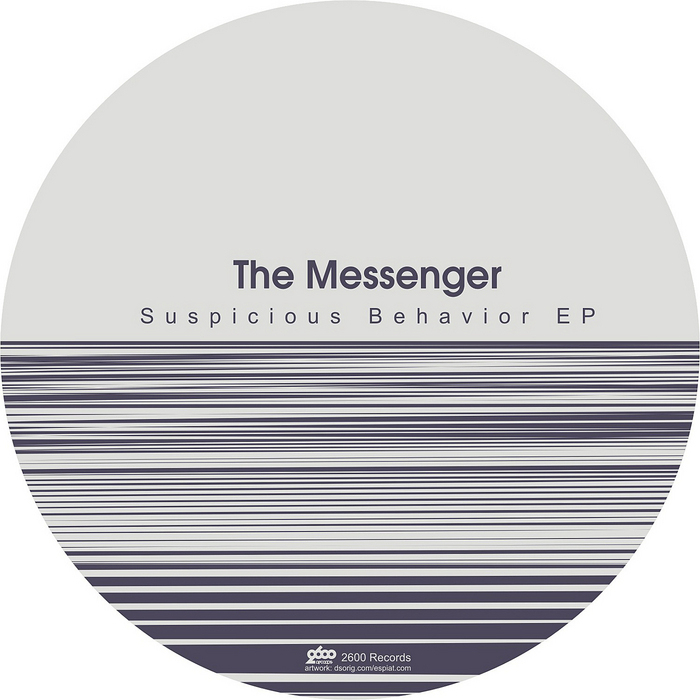
The configured features are then input into a variety of classification models based on SVM and KNN. These extracted features are first entropy coded, and then an ant colony system (ACS) is used to optimize the entropy-based coded features. Feature subset optimization is applied to the deep features that have been obtained. The dataset for detecting suspicious activity is subsequently sent to this pre-trained algorithm for feature extraction.
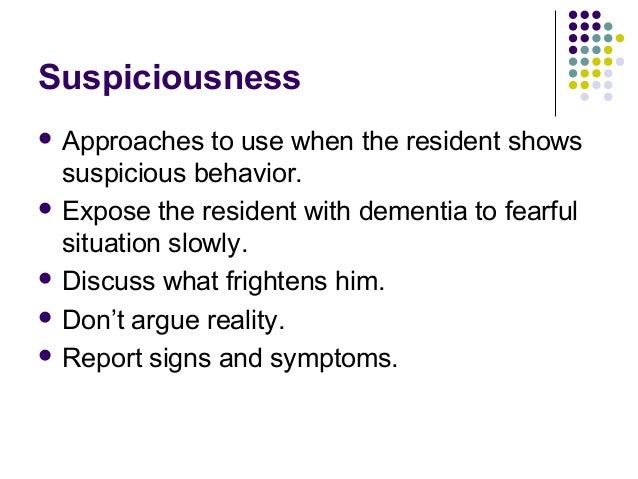
The developed framework is initially turned into a pre-trained framework by using the SoftMax function to train it on the CUI-EXAM dataset. The suggested CNN structure is centered on the alteration of VGG-16 with added four blanched. For this, a 63 layers deep CNN model is suggested and named "L4-BranchedActionNet". This work aims to distinguish the suspicious activities of students during the exam for surveillance examination halls.
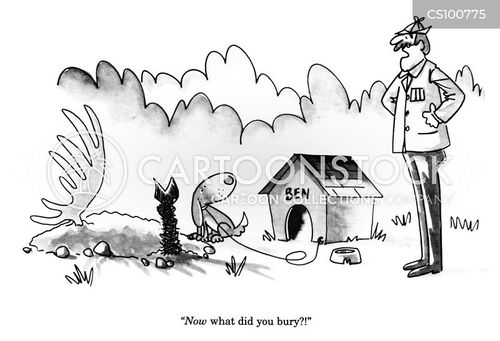
Know a day it is very possible to assure invigilators safety during the examination period. The existence of smart surveillance cameras with high processing power has opened the for making it conceivable to design intelligent visual surveillance systems. Video processing is getting special attention from research and industries.
